

Now when you add a third, fourth, or fifth variable, it’s harder to visualize on a graph like this in just two dimensions. And if that approach seems a little loosey-goosey to you, you could take a more scientific approach by measuring how close together the points are in one cluster and how far they are from points in other clusters to convince yourself that these clusters make sense. It is easy to visually pick out three clusters from this data, which I do below: people with little interest and a lot of concern (red), people with high interest and not much concern (green), and people who are a little bit of both (blue). For example, if the two variables were interest in owning an AV and concern about AV safety, we might expect the data to look something like this: It’s easiest to understand if we start by only considering two survey questions (variables). (See a great in-depth explanation on clustering here at Analytics Vidhya). These would be people who have similar attitudes about AVs. It looks for groups of observations that have more in common with each other than with others, by measuring distances between pairs of points. Clustering is a mathematical technique that works like this: I feed the computer all the data we have from the survey about AVs. With the data prepared, I turned to clustering. To prep the data, I removed blank values and converted worded answers to a numeric, ordinal scale to make them easier to analyze.
NERD CLUSTERS DRIVER
How interested are you in riding in an autonomous taxi, with a backup driver present?.How interested are you in riding in an autonomous taxi, with no driver present?.
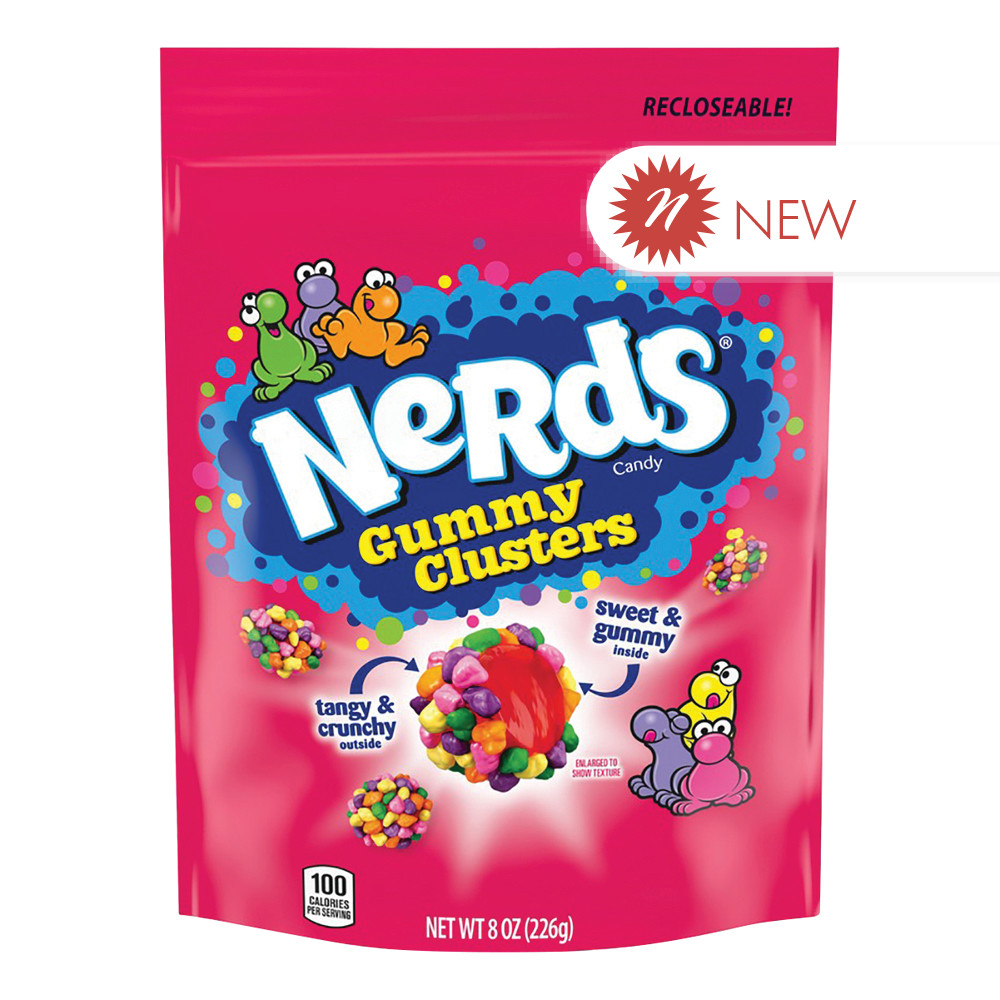
My data are comprised of ten questions assessing survey respondents’ interest in and concern about AVs.
NERD CLUSTERS FULL
As always, this is a summary but my full R code is on my Github. In this post, I’ll explain how I went about this analysis. Was everyone at one extreme or the other, or would I find nuance? I then used a technique called clustering to identify different types of people based on the answers they gave to survey questions about AVs. To help answer this question, I analyzed data from the Puget Sound Regional Council’s Household Travel Survey (2019) about residents’ attitudes toward this impending technological shift. How do people feel about autonomous vehicles (self-driving cars), really? Is everyone as vehemently opposed or totally gung ho as they seem? Are you the only one who hasn’t yet made up your mind? With new technologies, like many things, it can be hard to appreciate the nuance and broad range of feelings that people have. A cluster analysis suggests: actually a lot of us
